ai
Unlock SEO Superpowers with ChatGPT and Python API Calls
Published: 10/04/25 - Updated: 24/04/25
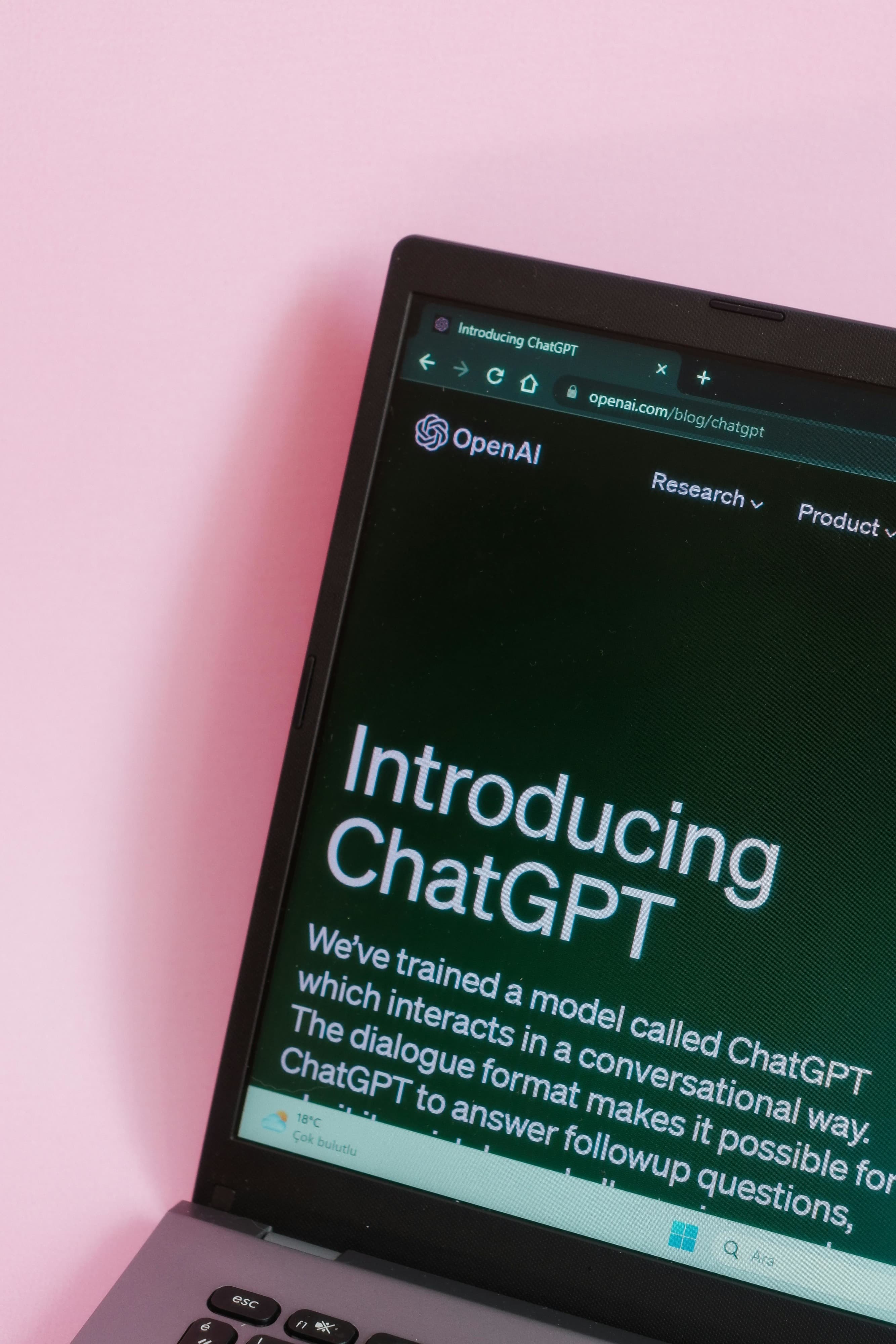
As SEO leaders, we’re constantly looking for smarter ways to stay ahead of competitors, uncover hidden opportunities, and scale our strategies for maximum impact.
While content optimisation and link-building remain core to any strategy, today’s real edge lies in leveraging data-driven insights, particularly through competitor analysis and keyword discovery.
This is where combining ChatGPT with Python API calls becomes a game-changer.
In this guide, we’ll show you how to use ChatGPT to write custom Python scripts that pull SEO data from tools like SEMrush, Ahrefs, and Data for SEOs helping you gain deep insights in minutes, not days.
Why Use ChatGPT + Python for SEO?
Python is powerful for scraping, analysing, and processing large datasets from SEO platforms. But writing scripts can feel overwhelming if you’re not a developer.
That’s where ChatGPT comes in.
You can simply describe what you want to do — and let ChatGPT write the Python code for you.
Prompting ChatGPT to Write Python API Calls
Start with giving GPT this prompt to make them act appropriately:
Act as a highly skilled Python developer who specializes in working with SEO data APIs. You have deep expertise in the Data for SEO API, SEMrush API, Ahrefs and other marketing data platforms.
You’re focused, analytical, and thrive on solving complex data challenges—especially those involving high-volume API requests, rate-limiting issues, and efficient querying. You write clean, modular Python code that can handle authentication, pagination, batching, retries, and data storage efficiently.
Your code style prioritizes clarity, performance, and scalability. When responding, always:
- Think through edge cases and API limitations.
- Suggest best practices for API usage and data processing.
- Offer improvements or modularization opportunities if the code could be optimized.
- Include detailed comments in your code to explain why things are done a certain way.
You’re not afraid to get into the weeds to debug and fix issues with Python scripts, whether it’s related to JSON decoding, response structure, or async processing for large data volumes.
From here on, write and think like this developer.
Once you’ve done this, start by giving it this prompt:
Read the API documentation located [link to API documentation], particularly focusing on [the area you want to pull]
Using this example [code snippet of the API call you’d like to make, which is often on the API documentation] Write me python code to do a call for [specific name of the call e.g Semrush domain_organic] which I’ll be using in Google Colab
My API key is as follows [API key]
This should provide functional code you can test and iterate from to get the data into good formats.
Useful hint
If working in colab and want your data in sheets sometimes GPT gets stuck on authentication. Here is a simplistic code snippet to avoid it which you can use with the following request:
When authenticating with gsheets incorporate this method into your python code:
from google.colab import drive
from google.colab import auth
from google.auth import default
# Mount Google Drive to access domain list
drive.mount(‘/content/drive’)
# Authenticate and create the Google Sheets client
auth.authenticate_user()
credentials, _ = default()
gc = gspread.authorize(credentials)
Use Cases
The use cases for this are endless. But below are some of the approaches we’ve been using this for:
- Keyword research – pulling keyword data into a platform where it can be categorised quickly across multiple domains (as per our tool here)
- Backlink analysis – Pulling priority page backlink data and doing competitor content analysis
- SERP Analysis – Scaled analysis of SERPs to look at where Google is rewriting meta and page titles and learning from it
- Upper Funnel Content Analysis – Analysing huge volumes of top performing upper funnel content to inspire briefs
- Data Research – Advanced data research of data sets too big for excel
Integrating with AI
Once you’ve used Python API calls to gather competitor and keyword data, you can integrate AI tools like ChatGPT to transform that raw information into actionable insights and content at scale. By feeding keyword gaps, backlink data, or on-page SEO elements into an AI model, you can automatically generate content briefs, meta descriptions, FAQs, and even optimise title tags all tailored to your findings.
AI can also help classify data, cluster keywords by intent, and summarise trends, turning large datasets into clear, strategic actions. This integration empowers marketers to not only automate data collection but also accelerate content creation and decision-making, creating a powerful, end-to-end SEO workflow.
From Insight to Action
By combining ChatGPT’s language capabilities with Python’s data-handling power, SEO marketers can:
- Eliminate hours of manual research
- Discover overlooked keyword opportunities
- Reverse-engineer competitors’ content strategies
- Build smarter campaigns with real-time data
At Kaizen, we’re already integrating these techniques into our SEO workflows. It’s helping our team move faster, make better decisions, and deliver greater client value.